Label Images for Object Detection: A Comprehensive Guide
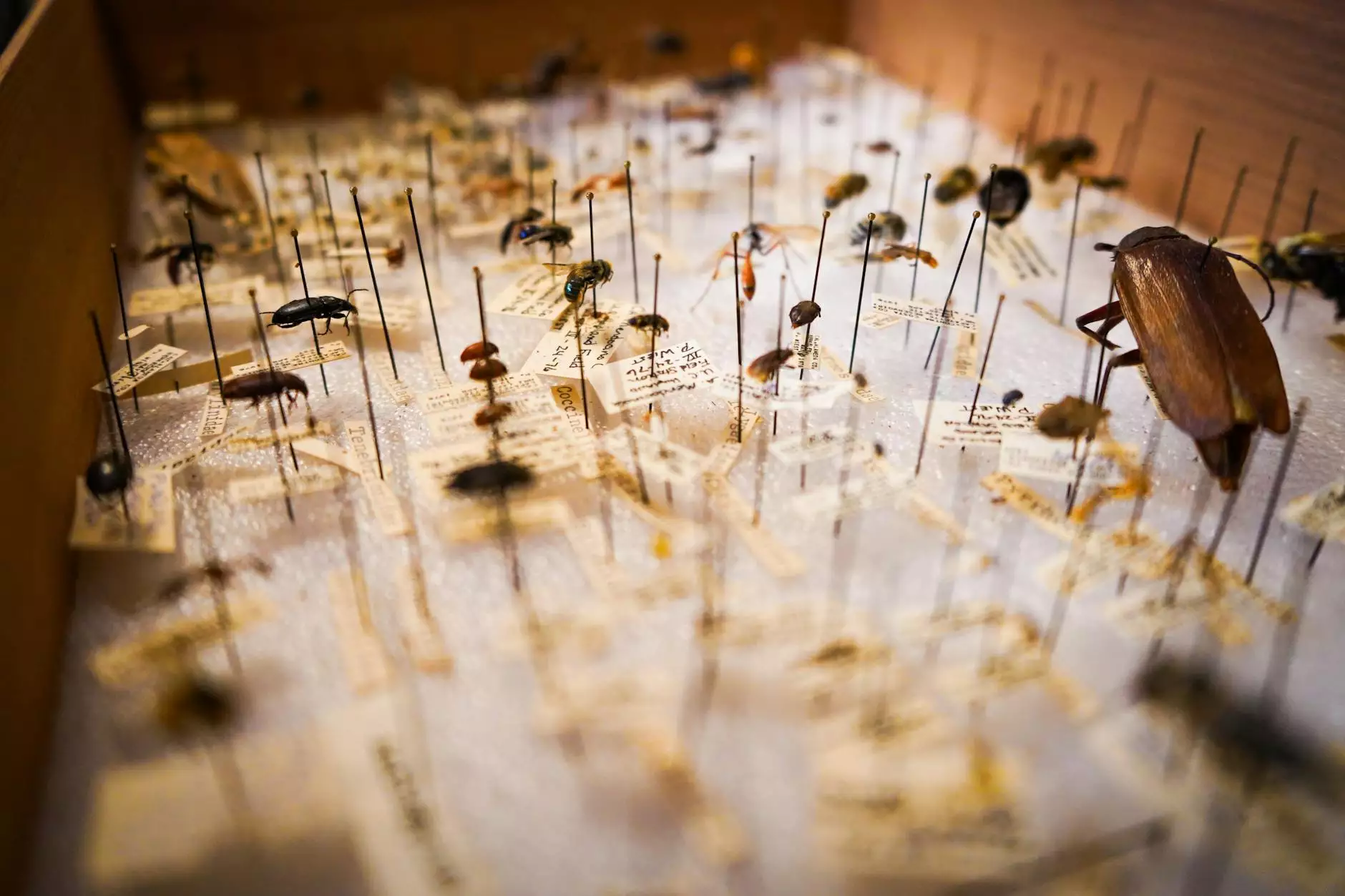
In the rapidly evolving world of technology and machine learning, the need for accurate and efficient data annotation is more crucial than ever. One of the primary tasks in training effective computer vision models is to label images for object detection. This article offers an in-depth exploration of how businesses can leverage advanced data annotation tools and platforms to maximize their operational potential and improve their machine learning results.
1. Understanding Object Detection
Before diving into the specifics of image labeling, it's important to understand what object detection entails. Object detection is a computer vision task that involves identifying and locating objects within an image. This technology is integral to various applications, from autonomous vehicles to surveillance systems.
1.1 The Importance of Object Detection in Business
Businesses across industries are incorporating object detection technologies to:
- Enhance Automation: Reduce the need for manual oversight through automated processes.
- Improve Accuracy: Achieve higher precision in identifying relevant items in various applications.
- Increase Efficiency: Streamline operations with faster processing times in data analysis.
- Foster Innovation: Create new products and services by leveraging advanced technologies.
2. The Role of Data Annotation
Data annotation is the process of labeling or tagging data to make it usable for training machine learning algorithms. In the context of object detection, this involves marking objects in images so that a model can learn to identify and classify them in new images.
2.1 Types of Data Annotation for Object Detection
When it comes to labeling images for object detection, there are several approaches:
- Bounding Boxes: This is the most common form of annotation, where a rectangular box encloses the object in the image.
- Segmentation Masks: Advanced annotation that outlines the exact shape of the object, providing pixel-level detail.
- Point Annotations: Used for specific objects where the exact boundaries are less critical.
- Polygons: Useful for irregularly shaped objects, allowing for more precise labeling than bounding boxes.
3. Choosing the Right Data Annotation Tool
Choosing the right data annotation tool is pivotal for businesses looking to optimize their machine learning projects. The ideal tool should be user-friendly, scalable, and integrate well with other platforms. Here’s how Keylabs.ai facilitates quality image labeling:
3.1 Keylabs.ai Data Annotation Tool
Keylabs.ai provides a robust platform tailored for efficient data annotation. Here are some key features:
- Intuitive Interface: Users can navigate easily, making the annotation process seamless.
- Automated Annotation Options: Leverage AI to speed up the labeling process while maintaining accuracy.
- Collaborative Tools: Team members can collaborate across projects, ensuring consistency and quality.
- Quality Control Mechanisms: Built-in checks and revisions to uphold high standards of annotation.
3.2 The Advantages of Using a Data Annotation Platform
Utilizing a dedicated data annotation platform like Keylabs.ai offers several advantages:
- Scalability: Handle large volumes of images quickly and efficiently.
- Cost-Effectiveness: Reduces operational costs by streamlining the annotation workflow.
- Expertise: Access to a team of skilled annotators as well as AI-driven tools to enhance output quality.
4. Best Practices for Labeling Images for Object Detection
To ensure the highest level of accuracy in object detection models, following best practices in image labeling is essential. Here are some guidelines:
4.1 Clear Labeling Guidelines
Establishing clear and concise labeling guidelines helps annotators understand the expectations and criteria for labeling. This includes:
- Defining Categories: Clearly categorize the objects to be annotated.
- Example Labels: Provide visual examples of correctly and incorrectly labeled data.
- Regular Updates: Ensure that the guidelines are updated as new objects or categories are introduced.
4.2 Quality Assurance Processes
Implementing quality assurance processes is crucial to maintain the integrity of the annotations. This can include:
- Double-Annotating Samples: Having multiple annotators label the same samples to compare results and resolve discrepancies.
- Regular Reviews: Frequent review and assessment of the annotated data to ensure compliance with guidelines.
- Feedback Mechanisms: Providing continuous feedback to annotators to refine their skills and improve future annotations.
5. Integrating Annotated Data into Machine Learning Models
Once the images are labeled, the next step is to integrate this annotated data into machine learning models. This involves training the model with the annotated datasets to enhance its ability to identify and categorize objects in new, unseen images.
5.1 Training and Validation
A well-structured training and validation process is essential. Here’s how to effectively manage this:
- Split the Dataset: Divide your annotated data into training, validation, and test sets to ensure balanced exposure.
- Monitor Performance Metrics: Track metrics such as precision, recall, and F1 score to evaluate model performance.
- Iterative Training: Continuously refine the model through iterative training cycles, improving accuracy with each round.
5.2 Deployment and Real-World Application
After training the model, it's critical to deploy it in a real-world setting effectively. Consider the following:
- Continuous Learning: Implement feedback loops where the model can learn from new data after deployment.
- Monitoring and Maintenance: Regularly monitor the model’s performance and adjust as necessary.
6. Future Trends in Object Detection and Data Annotation
As technology continues to advance, the landscape of object detection and data annotation is evolving. Here are some future trends to watch:
- AI-Powered Annotation Tools: Increased reliance on AI to automate and enhance the annotation process.
- Real-Time Annotation: Tools that allow real-time labeling capabilities during image capture.
- Augmented Reality Integration: Using AR for enhanced data visualization and annotation experiences.
Conclusion
In conclusion, the process of labeling images for object detection is not just a technical task but a foundational aspect of developing capable and accurate machine learning models. By utilizing advanced data annotation tools like those offered by Keylabs.ai, businesses can streamline their data practices, enhance their model's performance, and drive innovation in their respective fields. Investing in proper data annotation is an investment in the future of your business’s technological capabilities.