Maximizing Your Machine Learning Projects with Effective Labeling Tools for Machine Learning
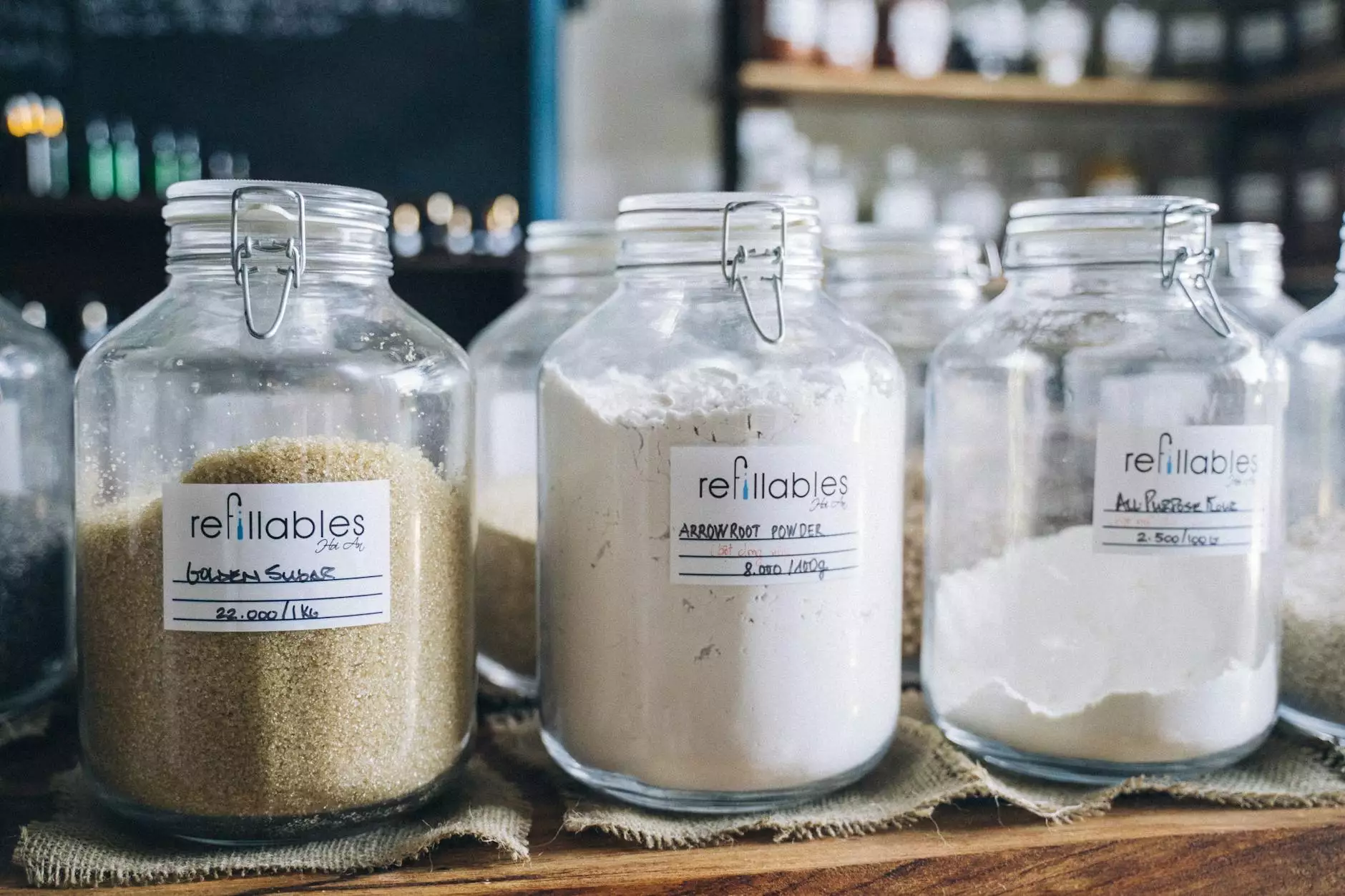
The rapid growth of machine learning in various industries has led to an increasing need for effective data management solutions. With the complexity of machine learning models, the quality of training data becomes critical. This is where labeling tools for machine learning come into play, providing businesses with the means to accurately annotate their data, which in turn enhances model performance and overall project success.
Understanding the Importance of Data Annotation
Data annotation is a fundamental aspect of the machine learning process. It involves the systematic labeling of data so that machines can understand and learn from the information provided. Here are various reasons why data annotation is vital:
- Enhances Model Accuracy: Properly labeled data improves the accuracy of machine learning algorithms, leading to better predictions.
- Facilitates Better Learning: High-quality annotations help algorithms learn more effectively, leading to improved model outcomes.
- Supports Diverse Applications: Annotated data can be used across different industries, from healthcare to finance, providing tailored solutions.
The Role of Labeling Tools in Machine Learning
Labeling tools for machine learning streamline the data annotation process. They offer various functionalities that enhance efficiency, accuracy, and collaboration. Some key aspects include:
1. Automation Capabilities
Many modern labeling tools for machine learning incorporate automation features that reduce the time required for manual annotation. This can involve pre-labeling based on existing data or leveraging artificial intelligence to suggest labels, thereby accelerating the annotation process significantly.
2. User-Friendly Interfaces
Top-tier labeling tools are designed with user experience in mind. Intuitive interfaces allow users, regardless of their technical expertise, to navigate the platform easily and contribute to the annotation process.
3. Collaboration Features
Machine learning projects often involve multiple stakeholders. Effective labeling tools provide collaboration features that allow teams to work together seamlessly, whether they're in the same location or spread across the globe. Real-time updates and communication channels ensure that everyone is on the same page.
Choosing the Right Labeling Tools for Machine Learning
When selecting labeling tools for machine learning, businesses must consider several factors that affect their project's success:
1. Types of Data Supported
Different projects require different types of data annotation. Some tools are optimized for image labeling, while others cater to text, audio, or video data. Ensure that the tool you choose aligns with your specific needs.
2. Scalability
Your labeling tool should be scalable to accommodate the growing size of your datasets. As your company expands, your tool should be equipped to handle increased demands without sacrificing efficiency or performance.
3. Customizable Workflows
Every project is unique. Look for tools that offer customizable workflows that can adapt to your specific labeling needs and processes.
Highlighting KeyLabs.ai: A Top Choice for Data Annotation
At KeyLabs.ai, we understand the intricacies involved in data annotation and the pivotal role of labeling tools for machine learning. Our solutions are designed with the following features:
Comprehensive Annotation Support
KeyLabs.ai provides robust support for various data types, ensuring flexibility for your projects. Whether you are working with images, text, or videos, our tools are equipped to handle complex annotation tasks.
Advanced AI Integration
Our labeling tools leverage the latest in artificial intelligence to facilitate pre-annotation and enhanced label suggestions. This reduces time spent on manual entry and increases overall annotation quality.
Simplified Collaboration
With features designed for team collaboration, KeyLabs.ai ensures that your stakeholders can easily communicate, share insights, and contribute across the annotation process efficiently.
Best Practices in Data Annotation Using Labeling Tools
To maximize the efficiency of labeling tools for machine learning, consider implementing the following best practices:
1. Define Clear Guidelines
Establishing clear annotation guidelines helps maintain consistency throughout the project. Ensure that all annotators understand the project’s goals and the criteria for labeling.
2. Regular Quality Checks
Conduct regular quality assurance checks to identify discrepancies and provide feedback to annotators. This can improve accuracy and ensure high-quality results.
3. Train Your Annotators
Investing in training for your data annotators can dramatically improve the quality of the annotations produced. This includes familiarization with the labeling tool, understanding the dataset, and refining annotation skills.
The Future of Labeling Tools in Machine Learning
The evolution of labeling tools for machine learning is ongoing, with advancements in AI and machine learning continuing to shape the landscape. Here are some trends to watch for:
1. Increased Automation
As AI technology matures, expect automated labeling capabilities to become more sophisticated, enabling even greater reductions in manual work while maintaining accuracy.
2. Enhanced AI-Assisted Labeling
Future labeling tools will likely include even more powerful AI-driven features that assist annotators by suggesting corrections or automatically categorizing data based on learned behaviors.
3. Broader Integration
The integration of labeling tools with other machine learning platforms and services will streamline workflows, making it easier to manage projects from data collection to deployment.
Conclusion
In the landscape of machine learning, the importance of labeling tools for machine learning cannot be overstated. They play a crucial role in the success of data annotation, which is vital for training algorithms effectively. KeyLabs.ai provides cutting-edge solutions designed to enhance the quality, speed, and collaboration of the annotation process, ensuring that your machine learning projects yield optimal results. Embrace the future of data annotation with KeyLabs.ai and take your machine learning initiatives to the next level.