Unlocking the Potential of Data Labeling in Machine Learning
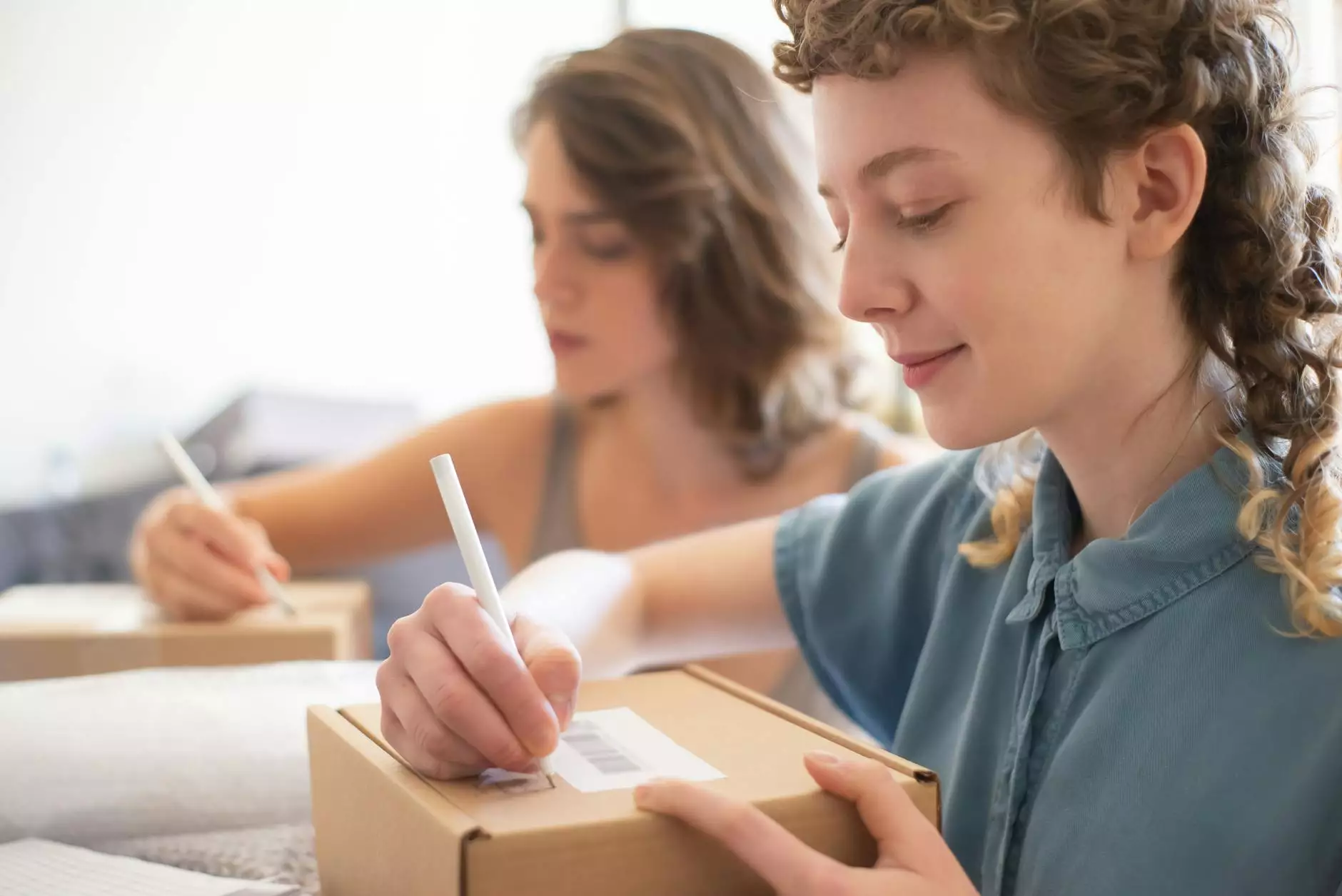
In today's digital landscape, machine learning has emerged as a transformative technology that is set to reshape industries and redefine efficiency. However, the effectiveness of any machine learning model lies significantly in its foundation: the data it is trained on. This brings us to the crucial concept of data labeling. Understanding data labeling is essential for businesses that are looking to leverage machine learning for their benefit.
What is Data Labeling?
Data labeling is the process of tagging or annotating data so that machine learning algorithms can learn from it. This can involve anything from identifying objects in images to transcribing audio files and classifying text documents. The better the quality of the labeled data, the better the performance of the machine learning models.
The Importance of Data Labeling in Machine Learning
Without high-quality labeled data, machine learning models cannot achieve their full potential. Here are several reasons why data labeling is crucial:
- Enhances Model Accuracy: Properly labeled data is vital for training accurate machine learning models. Without it, the models may make incorrect predictions or classifications.
- Facilitates Data-Driven Decisions: Businesses that utilize machine learning can make more informed decisions based on data analysis, leading to enhanced strategies and outcomes.
- Supports Diverse Applications: Data labeling is applicable across various fields, from healthcare to finance, making it a versatile solution for many industries.
- Improves User Experience: High-quality data labeling leads to better AI applications, providing users with more precise recommendations and experiences.
Types of Data Annotation
Data annotation can be categorized into different types based on the data format and the nature of the tasks involved. Here are the primary types:
1. Image Annotation
This involves labeling images for various purposes, such as object detection, image segmentation, or facial recognition. The types of image annotation can include:
- Bounding Box Annotation: Drawing boxes around objects of interest in an image.
- Polygon Annotation: Outlining objects with a polygon shape for more accurate segmentation.
- Landmark Annotation: Marking specific points on images, such as facial features.
2. Text Annotation
Text annotation involves the process of labeling textual data for sentiment analysis, named entity recognition, and more. Common methods include:
- Named Entity Recognition: Identifying and categorizing key entities in text such as names, organizations, and locations.
- Sentiment Analysis: Classifying text based on sentiment, whether positive, negative, or neutral.
- Part-of-Speech Tagging: Labeling words in text with their corresponding part of speech.
3. Audio Annotation
Audio annotation is used for labeling audio files, usually in applications such as speech recognition or audio classification. The main types include:
- Transcription: Converting spoken language into written text.
- Sound Recognition: Identifying and labeling different sounds or speakers in an audio track.
Why Choose KeyLabs.ai for Data Annotation?
When it comes to selecting a data annotation platform, KeyLabs.ai stands out as an industry leader. Here’s why:
1. Advanced Data Annotation Tools
KeyLabs.ai provides a suite of data annotation tools that are designed to streamline and enhance the labeling process. These tools are equipped with features designed for accuracy and efficiency:
- Customizable Interfaces: Tailor the user experience to fit specific project needs.
- Batch Annotation: Save time by annotating multiple data points simultaneously.
- Automated Suggestions: Leverage machine learning to aid in the annotation process, reducing manual effort.
2. Human-Centered Annotation Process
At KeyLabs.ai, we understand the importance of human insights in data labeling. That’s why our platform combines automated tools with a skilled workforce that ensures the highest quality annotations.
3. Scalability
Whether you have a small dataset or need to annotate massive amounts of data, KeyLabs.ai can scale to meet your needs without compromising on quality or speed.
Business Benefits of Data Annotation
Investing in data annotation can yield numerous benefits for businesses looking to adopt machine learning solutions:
1. Increased Efficiency
By utilizing data annotation tools and platforms like KeyLabs.ai, businesses can significantly reduce the time and resources spent on preparing data for machine learning models.
2. Cost Reduction
Effective data annotation can lead to long-term cost savings. Accurate models reduce the likelihood of errors, minimizing wasted time and resources that may result from bad data-driven decisions.
3. Enhanced Competitiveness
Companies that invest in properly labeled data gain a competitive edge. Enhanced predictive capabilities lead to better product offerings and customer satisfaction.
4. Data Privacy and Compliance
KeyLabs.ai is committed to maintaining data security and compliance with regulations, which is crucial as businesses navigate an increasingly complex data privacy landscape.
Challenges in Data Labeling
While data labeling is essential, it is not without its challenges. Some of the common issues companies face include:
1. Time-Consuming Process
Data labeling can be labor-intensive and time-consuming, especially large datasets. Solutions that reduce annotation time are crucial.
2. Subjectivity
Human annotators may have varying interpretations of data, leading to inconsistencies. It’s vital to have thorough guidelines and quality checks in place.
3. Scaling Difficulties
As project scopes grow, maintaining quality at scale can be challenging. Advanced tools and platforms like KeyLabs.ai are essential in such scenarios.
The Future of Data Labeling and Machine Learning
The landscape of machine learning is evolving rapidly. As we move forward, we can expect the following trends to shape the future of data labeling:
- Increased Automation: More tools will integrate AI to automate parts of the labeling process, enhancing speed and accuracy.
- Greater Demand for Diverse Datasets: As machine learning expands into more sectors, the need for varied and abundant labeled datasets will grow.
- Focus on Ethical AI: As data privacy regulations become stricter, ethical considerations in data labeling will gain more attention.
Conclusion
In conclusion, the role of data labeling in machine learning cannot be overstated. By investing in efficient data annotation tools and platforms like KeyLabs.ai, businesses can unlock the full potential of their machine learning initiatives. Whether you aim to enhance operational efficiency, improve customer experiences, or stay ahead in a competitive market, quality data labeling is the cornerstone of success. Embrace the future of machine learning with robust, accurate, and high-quality labeled data.
For more information on data annotation and how KeyLabs.ai can help streamline your data labeling process, visit keylabs.ai.
data labeling machine learning